Testing Token Efficiency and Response Behavior: Comparing LLaMA and DeepSeek
I personally used the Groq API to test and compare how two AI models perform with the same input. I compared two powerful models—LLaMA 3 70B Versatile and DeepSeek R1 Distill LLaMA 70B—by sending an identical JSON prompt requesting Selenium Java code for Salesforce login automation. My goal was to analyze and test how these models handle the same task in terms of token consumption, response time, and output clarity within the Groq platform.
The results were insightful.
Llama response:
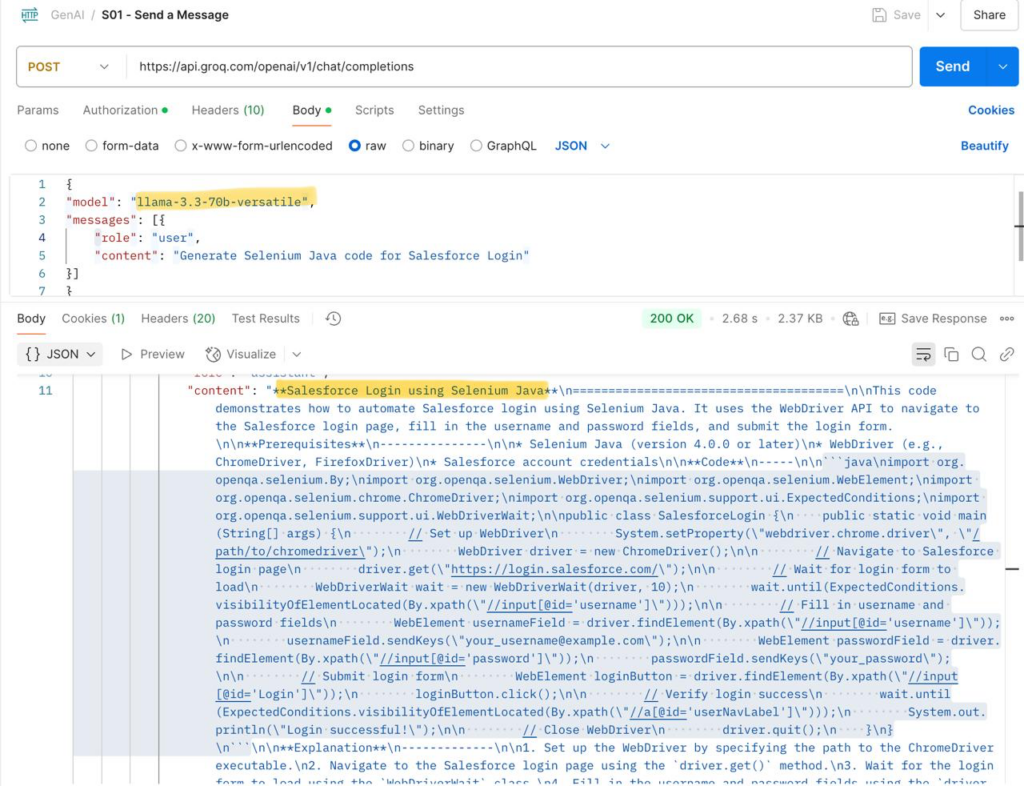
“usage”: {
“queue_time”: 0.20843550800000002,
“prompt_tokens”: 42,
“prompt_time”: 0.002330543,
“completion_tokens”: 598,
“completion_time”: 2.174545455,
“total_tokens”: 640,
“total_time”: 2.176875998
}
Deepseek response:
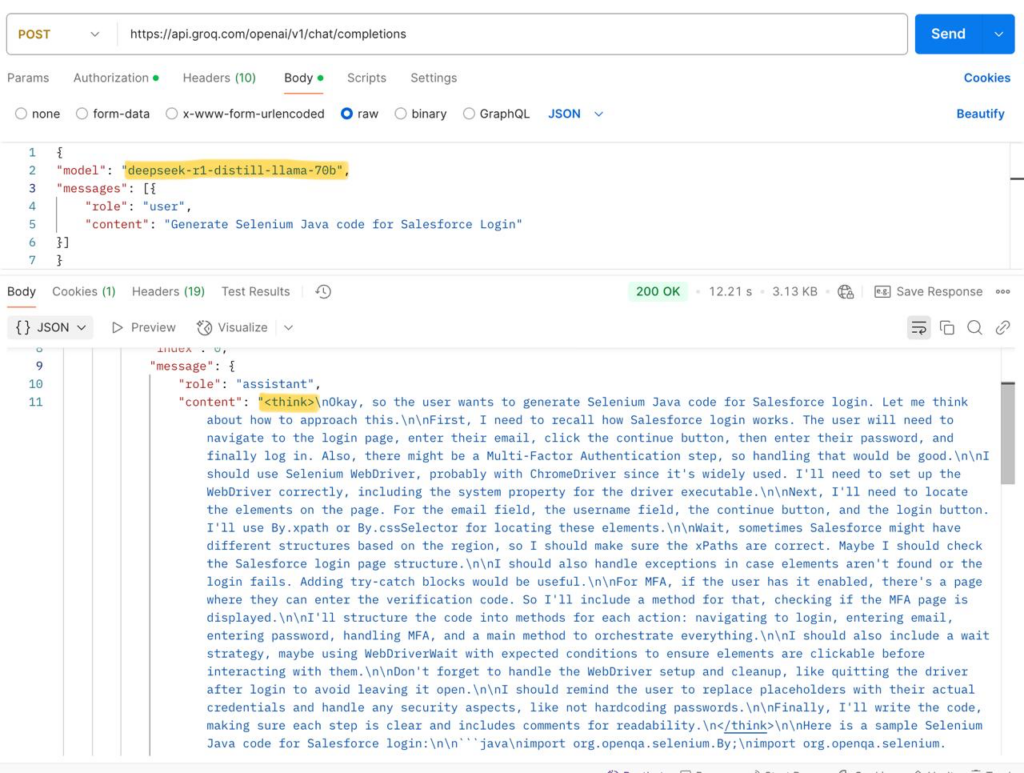
usage”: {
“queue_time”: 7.729306837999999,
“prompt_tokens”: 10,
“prompt_time”: 0.003810801,
“completion_tokens”: 1176,
“completion_time”: 4.276363636,
“total_tokens”: 1186,
“total_time”: 4.280174437
}
The results were insightful. The LLaMA 3 model processed a total of 640 tokens and returned a detailed code snippet in just 2.17 seconds, with minimal queue time. In contrast, DeepSeek consumed 1186 tokens, taking about 4.28 seconds, with a noticeably longer queue time of 7.72 seconds. Interestingly, while DeepSeek included internal thinking steps and reasoning in its response :), LLaMA focused on direct code generation with clearer formatting.
This exploration highlights how token usage and response structure can vary significantly even with the same prompt. For developers and testers integrating AI models via Groq API, understanding these differences is key to choosing the right model for speed, precision, and contextual output style. I look forward to sharing further comparisons as I continue experimenting with prompt structures, execution logs, and advanced QA use cases.