Optimizing AI- Why Fine-Tuning Parameters Is Key to Better Results
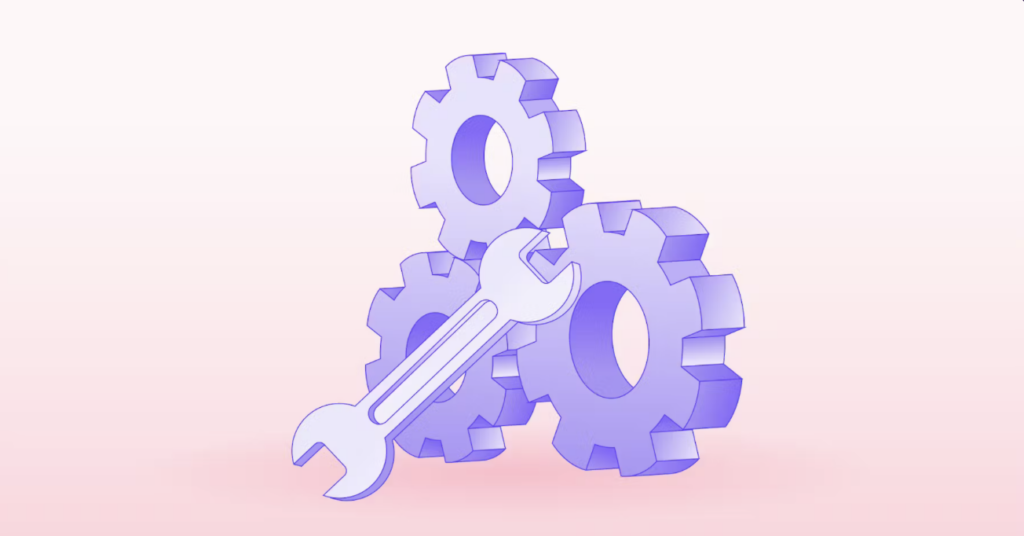
Image Credit: Encord
Fine tuning parameters in AI models is more than adjusting dials- it’s about aligning the model’s behavior with real world need. When dealing large and dynamic datasets, especially in industries like retails, the right parameter tuning ensures that outputs are not accurate but context aware. Instead of relying on default settings, adjusting values like model-specific scoring weights allows to control, how the model responds to different scenarios, balancing consistency, creativity and efficiency. This level of control is especially important when input data reflects changing patterns or seasonal behavior.
For example let’s take a retail giant like Walmart as an example. Throughout the year, their business is driven by seasonal trends like Black Friday, Back to school, Holiday sales and more. Each season introduces different factors that influence which product sells and why. Key parameters like Product availability, price, discount levels, inventory, brand value, shelf space and promotional. visibility all play a role. But during a high traffic like a seasonal sale, certain attributes carry more weight – availability, shelf presence, and discount rate many need more emphasis than brand. By fine tuning the weights of these parameters in an AI powered recommendation or forecasting model, Walmart can ensure its decision making aligns with what matters most at that moment.
In the same way, when we fine tune parameters in AI systems- whether for testing strategies, generative output or predictions- we are giving the model context. We are telling it what to prioritize, when and why. This becomes especially powerful when datasets are large, noisy, or even changing frequently. By fine tuning we can guide the model’s focus toward business critical insights, reducing noise and maximizing relevance. It transforms AI from a general purpose assistant to a precision aligned partner and delivering results that fit for the business goals- not just statistically but strategically.