Understanding How Temperature Parameter Controls AI Behavior in LLMs
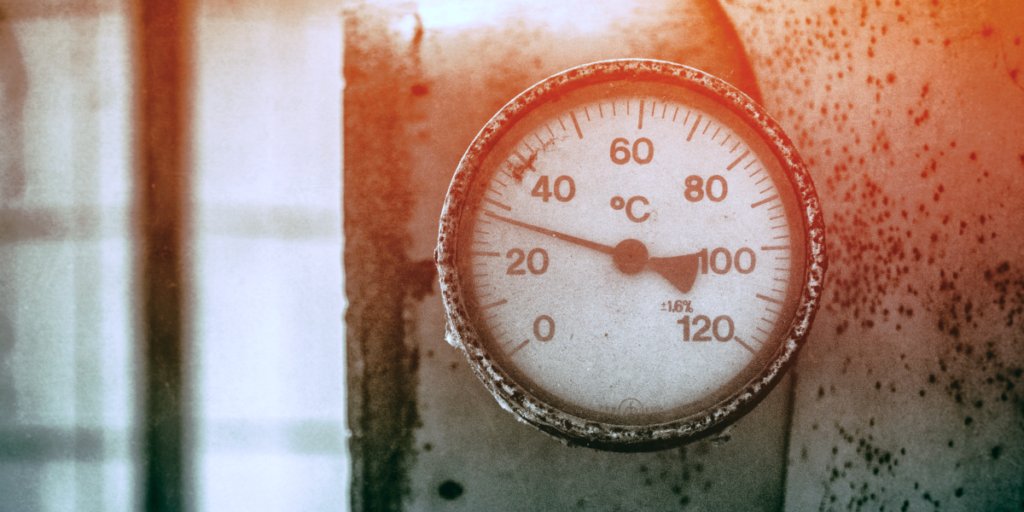
Image credit: statsig
Fine tuning the behavior of an AI model is not just about writing the right prompt-It is also about adjusting the temperature behind the scenes. In LLMs temperature controls how creative or focused the model’s output will be. A lower temperature such as 0.0-0.2 keeps the response consistent, fact based and deterministic, which is ideal when you need structured outputs like Json, code generation or technical facts. A higher temperature closer to 0.9-1.5 allows the AI to become more imaginative and diverse, generating unique, creative ideas perfect for brainstorming or marketing content.
Depending on the use case, choosing the right temperature is crucial. If you are generating technical documentation or writing tutorials a moderate temperature around 0.3-0.5 keeps the output slightly flexible while maintaining structure. For chatbots and support agents, a slightly higher temperature 0.6-0.8 ensures the conversations feel more natural without becoming too random. For creative writing task like story development or idea generation, pushing the temperature between 0.9-1.2 encourages diverse and fresh outputs. Finally, for marketing and advertisement, where highly engaging and unique content is key, so the temperature can be set as higher value like 1.2-1.5.
Understanding how to adjust the temperature empowers you to guide AI behavior based on our goals- whether that’s precision, creativity or natural conversation. As shown in the use case examples, turning temperature allows QA testers, developers, content creators, and strategist to get outputs the fit their specific needs. By mastering this one simple parameter, you can turn an AI model into a more reliable assistant, creative partner or anything in between- just by moving single dial 🙂
Note:
The temperature ranges and settings shared in this blog are intended as general guidelines based on common use cases. In real-world applications, the ideal temperature value may vary depending on your specific model behavior, business needs, and context. It is always recommended to experiment and fine-tune based on your goals, desired output style, and quality expectations.